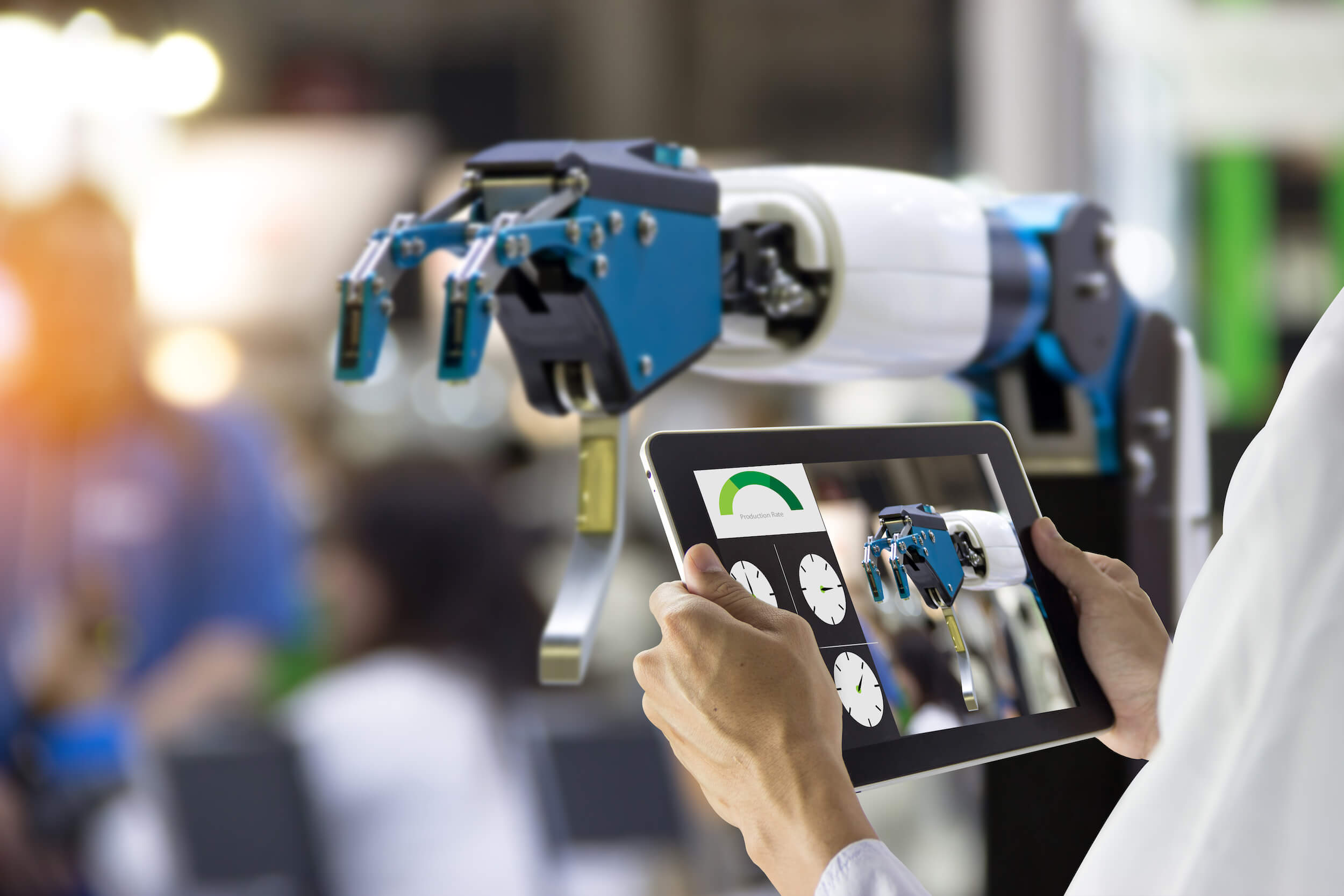
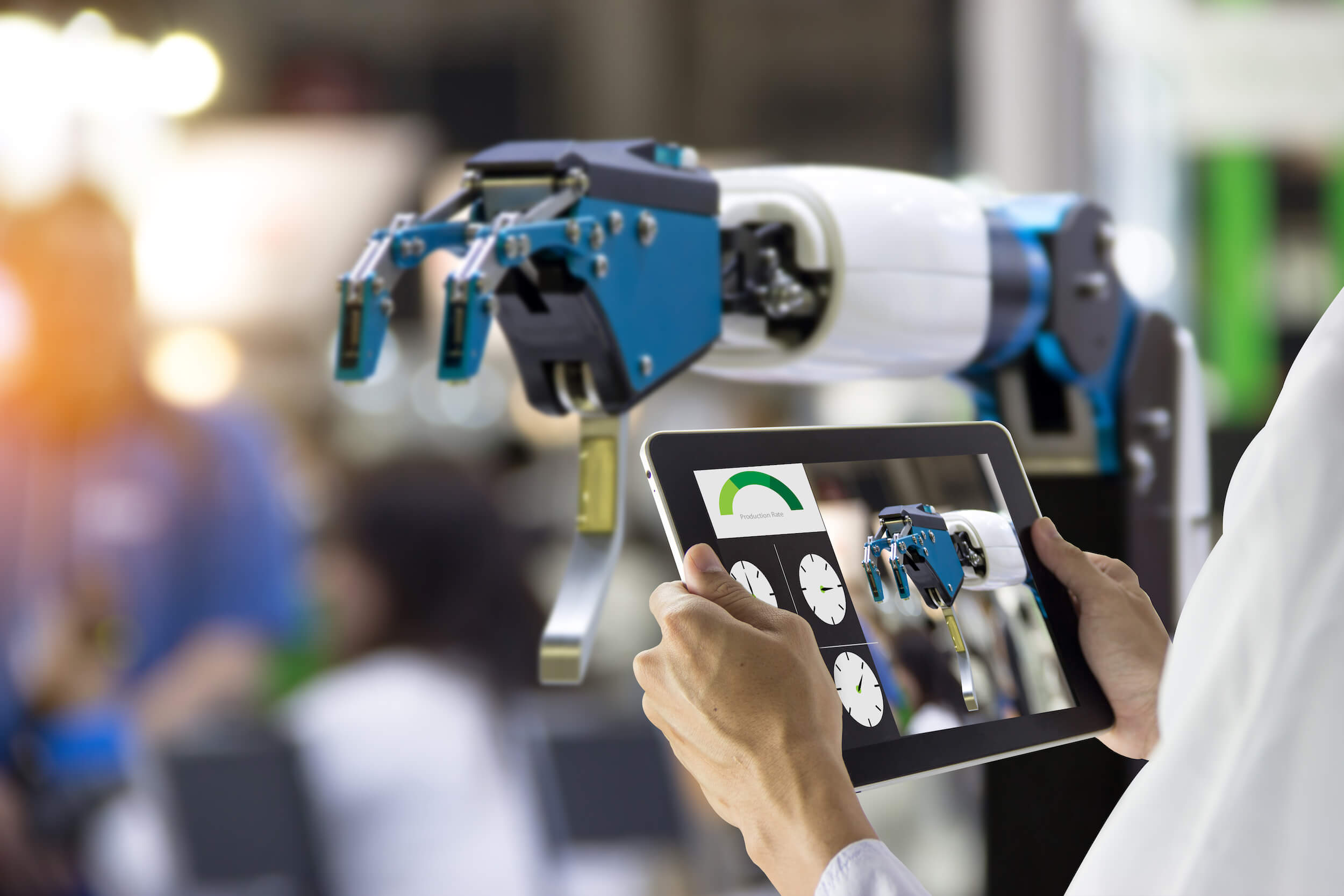
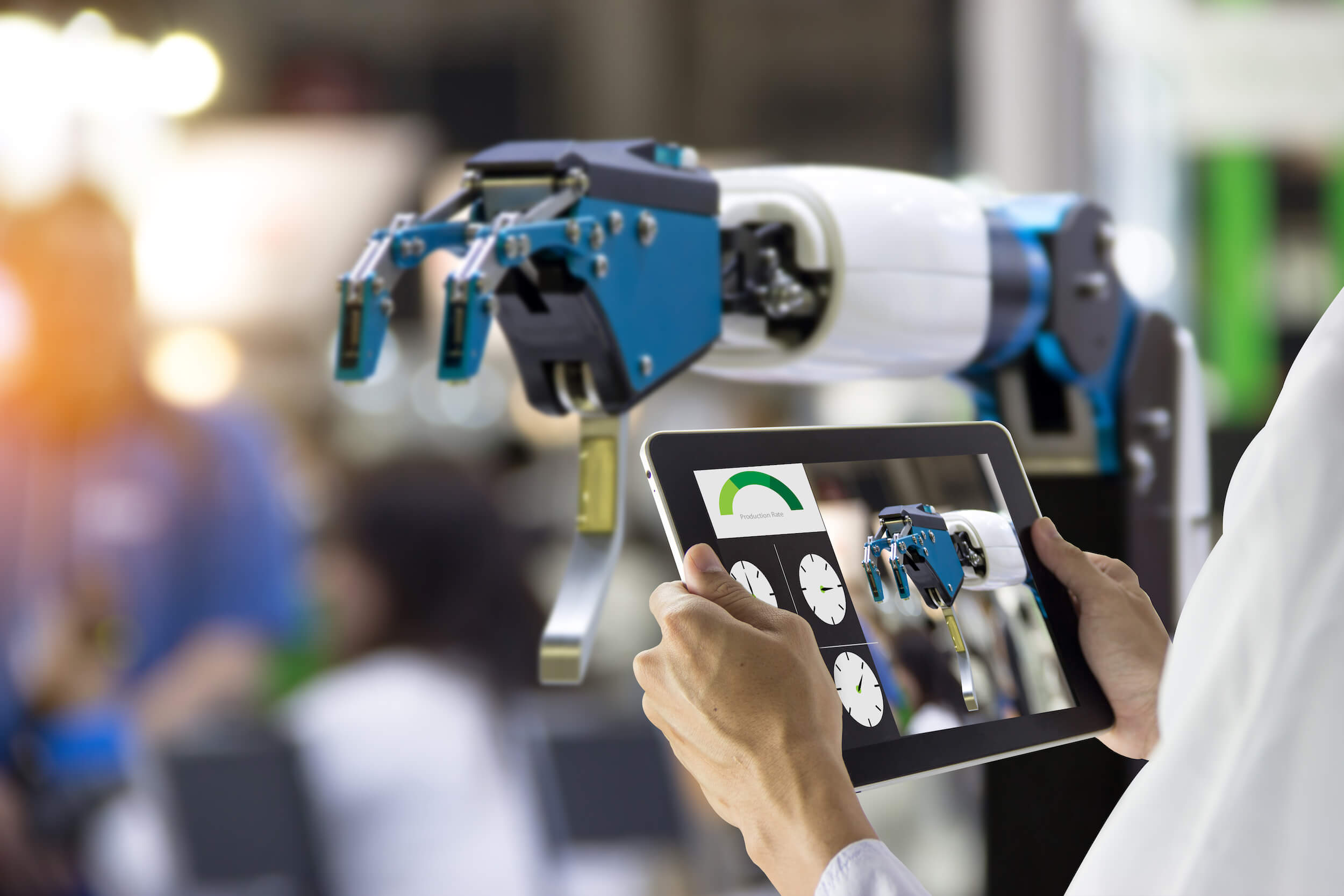
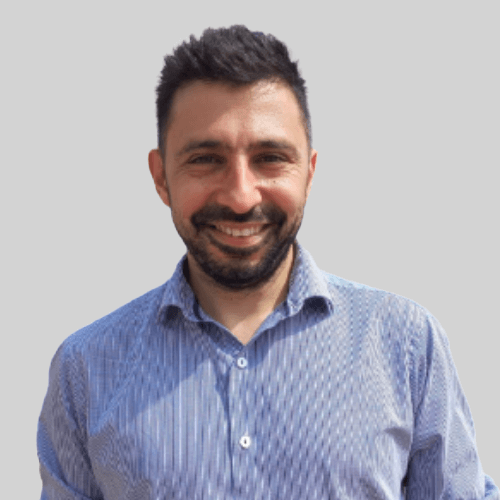
"Every now and then something happens—ultimately linked to changes in industrial technique, although the connection is not always obvious—and the whole spirit and rhythm of life changes, and people gain a new perspective that is reflected in their political behaviour, their manners, their architecture, their literature and everything else." George Orwell, 1942
The world of work, disrupted
George Orwell’s description of deep social transformations and their undeniable connection with arising production methods sound very actual. The goods and services that we produce, trade, and consume—and the way we do it—are changing fundamentally thanks to a confluence of a diverse set of organisational and Information and Communication Technologies, with artificial intelligence (AI), cloud computing, and the proliferation of data-driven decision-making businesses leading the way (see NASEM, 2020).
Disruptive technologies are key to the labour market because they have the ability to redefine the world of work entirely. The Neolithic Revolution transformed hunter-gatherers into farmers; the Industrial Revolution converted the self-employed into factory workers (Lucassen, 2022). In many cases, new labour relations came with the movement of workers from low-productivity jobs to high-productivity jobs, resulting in higher economic growth and unprecedented improvements in living standards.
How can societies take advantage of the transitions to close these gaps and promote quality jobs? In the last five years, many research efforts by researchers worldwide and multilateral agencies have been oriented to develop conceptual schemes to answer this question (see for example, World Bank, 2019; IMF, 2018; and OECD, 2019). Very schematically, there are, on the one hand, the drivers of change and, on the other hand, the collective responses of society, organises basically through public policies and regulations. Among the drivers of change, the one that appears most frequently is technological change, led by digitalisation and AI. Demographics, environmental issues, globalisation and migration are also mentioned in varying degrees. Concerning public policy responses, human adaptability is measured mainly through changes in learning systems in their different instances: early childhood, formal education, and technical and vocational training. A second line of response comprises two types of policy: those associated with labour institutions, focusing on social protection, and those related to technology and innovation policy, focusing on directing technological innovation towards sustainable and inclusive development. Finally, another set of policies (migration, international trade, family) also appears in some schemes.
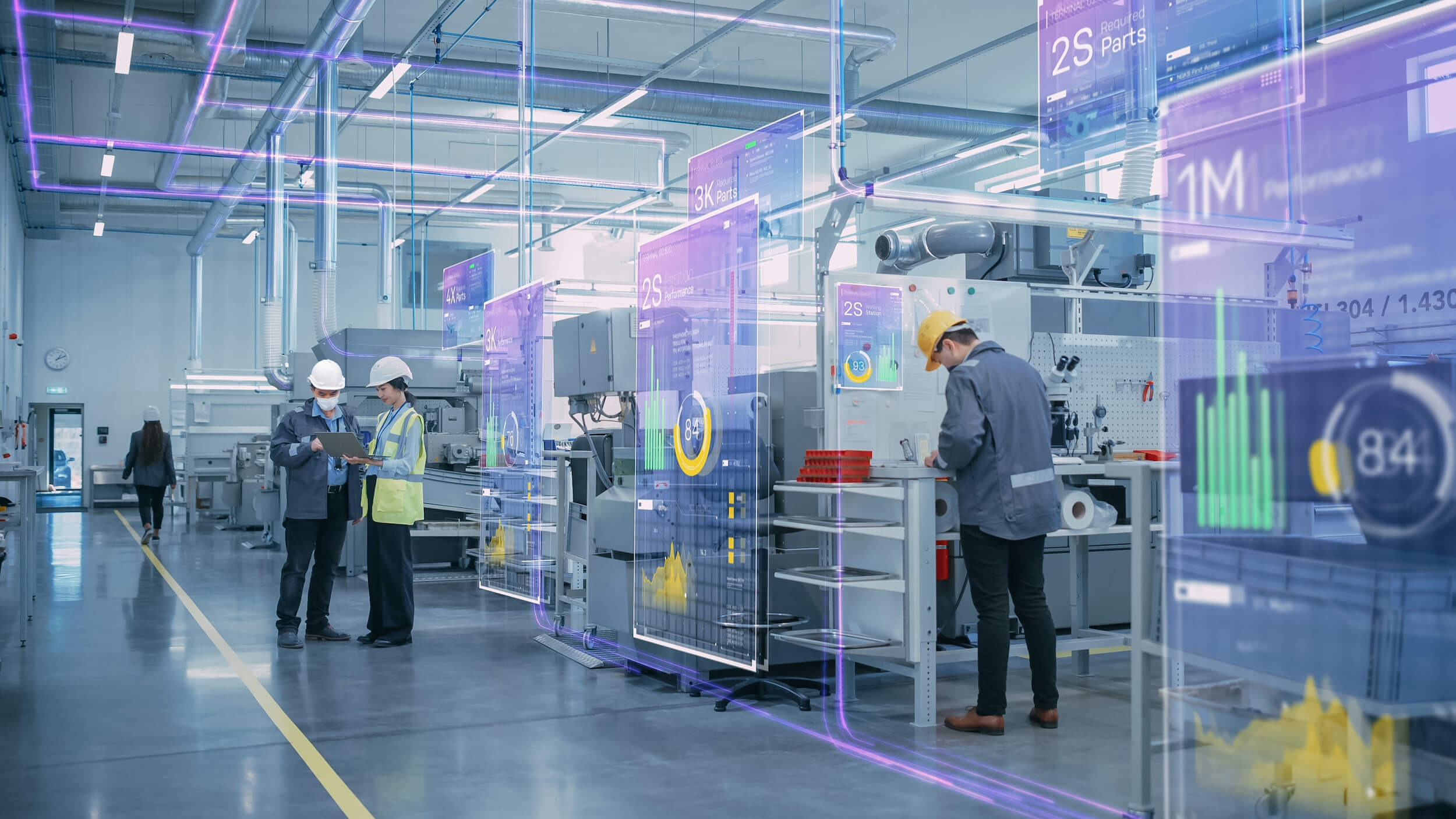
The reskilling challenge
Let’s focus on the primary policy response: education and skills.
We have yet to determine what the set of skills will be required in the future labour market amid the AI revolution. What we do know is that the challenge for people is to stop acting like robots. When Karel Capek invented the word “robot” in his 1921 science fiction play, he thought of it in a very different sense than we use it today. It did not refer to machines that managed to recreate the human cognitive ability fully; instead, Capek used it to describe how dehumanised jobs were in the era of the big factory and mass production (see Rhee, 2018). A century later, it seems that little has changed: when in 2015 the physicist César Hidalgo was asked if machines could think, he retorted: “the key question is whether we are training people who have the ability to think.” (Brockman, 2015).
What does this “ability to think” mean in the current context? With the emergence of AI and the creation of data-rich environments, a new division of tasks began to emerge in the production process, and with this, a new partnership between machines and people.
In this new division of tasks, the broad and general set of skills that used to be sufficient to guarantee workers a place in the middle class is no longer enough. Tasks such as making transactions, performing repetitive manual tasks, engaging in data-driven predictions and other highly routinised activities reflect Capek’s criticism and can be performed more efficiently and economically by AI-powered machines.
The first set of skills that were considered less important and difficult to stimulate, but came to have a central role in the new paradigm are those typically encompassed under the term soft skills. According to UNESCO, examples of soft skills are empathy, communication, motivation, flexibility, a sense of responsibility, and time management, among others.
A second set of skills that gained relevance is associated with understanding the generality and purpose of the tasks that make up the production process. In a sense, it has to do with the return of artisan work: non-standardised products adapted to the specific objectives of each particular case. But it is also about acquiring a holistic view of the processes in order to detect how the different parts of a whole are related. For an AI system to succeed, it is necessary to know precisely all the tasks of the production process, how these tasks are connected in different contexts, and subset of them that can be represented as a database. In this case, foundational skills, such as reading and basic mathematics, are critical.
Lastly are the skills required to interact with machines known as digital skills. In this regard, two sets of skills are of relevance. First, people help the system to function properly by complementing the machines. For example, in the training stage, the generation of databases is key, which is less a simple extraction of pre-existing information than obtaining, cleaning, structuring, testing, and correcting a broad set of digital fingerprints. For the systems to be free of biases or comment on errors, the databases generated must achieve a correct representation of the environment, and in this objective, people are essential. Second, as AI has the ability to increase the cognitive capacities of humans, new skills are needed, particularly for reimagining processes and products given these new technologies, and for designing the architecture to take advantage of them.
In what stages of life are these skills forged? Recent advances in neuroscience inform us that the relationship between biology and the environment is a two-way process: biology delimits our interactions with the environment, but the environment also affects biological development. In particular, today, it is possible to more accurately detect critical and sensitive periods where external stimuli affect cognitive development. For example, Gerry Leisman and his colleagues conclude that there are two particularly sensitive periods: early childhood and adolescence (Leisman et al., 2015). Thus, any assessment of the skills necessary for the 21st century must focus on three learning processes: early childhood (where soft skills are primarily forged), adolescence (where others related to greater abstraction and digital nature are learned), and people who are already in the job market and who must adapt what they have already learned and, at least in the margin, incorporate new knowledge.
The ASEAN response
A quick look at ASEAN countries shows that there’s room for improving the set of skills development policies and institutions.
Concerning soft skills, globally, six out of ten children are enrolled in the pre-primary education system, a ratio that rises to 85 per cent in the subset of high-income countries. In ASEAN countries, the picture is heterogeneous because the region includes lower-middle-income, upper-middle-income, and high-income countries. Thus, in Thailand or Singapore, the enrollment rate in pre-primary education exceeds 75 per cent, while in lower-income countries—such as Cambodia or Myanmar—it falls below 40 per cent. In terms of spending per student, the world spends about 2,300 US dollars per year in PPP terms and ASEAN countries much less (about 1,200 US dollars in Malaysia and about 670 US dollars in Brunei Darussalam).
With respect to foundational skills, globally, almost six out of every ten children who complete primary education reach a minimum level of proficiency in text comprehension. Several countries in the region are close to this average. Still, in the vast majority of countries —particularly those with lower-middle incomes—this ratio drops dramatically to one or two in ten. Learning performance in mathematics paints the same picture.
What do we know about digital skills? Here the available evidence is scarce. However, we know that globally about one in four people over the age of 15 knows how to use a spreadsheet on a digital device. The gap within ASEAN countries is very large: from 42 per cent in Brunei to 9 per cent in Cambodia. We also know that less than one in twenty adults globally knows how to write a computer program. In ASEAN, there are countries where this ratio is significantly higher—such as Brunei, Malaysia or Singapore—and others where this percentage is close to 1 per cent (such as the Philippines).
A challenging agenda
In sum, the ASEAN region has a significant challenge ahead. AI and other technologies represent an opportunity to accelerate growth and generate more and better jobs. But lower-income countries have a huge challenge in terms of reforming education systems and reskilling. Expanding coverage in early childhood systems, improving the quality of learning in formal education, and a more ambitious policy in learning digital skills for the population must be the three pillars of a skills development system that aims to transform the AI opportunity into a reality.